목록논문 (10)
while (1): study();
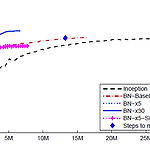
출처: https://arxiv.org/abs/1502.03167 Batch Normalization: Accelerating Deep Network Training by Reducing Internal Covariate Shift Training Deep Neural Networks is complicated by the fact that the distribution of each layer's inputs changes during training, as the parameters of the previous layers change. This slows down the training by requiring lower learning rates and careful param arxiv.org 지..
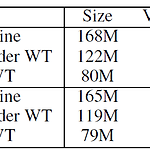
출처: https://arxiv.org/abs/1608.05859 Using the Output Embedding to Improve Language Models We study the topmost weight matrix of neural network language models. We show that this matrix constitutes a valid word embedding. When training language models, we recommend tying the input embedding and this output embedding. We analyze the resulting upd arxiv.org * 하단 포스팅의 후속 포스팅입니다. https://jcy1996.tis..
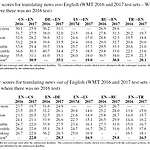
출처: https://aclanthology.org/W17-4739/ The University of Edinburgh’s Neural MT Systems for WMT17 Rico Sennrich, Alexandra Birch, Anna Currey, Ulrich Germann, Barry Haddow, Kenneth Heafield, Antonio Valerio Miceli Barone, Philip Williams. Proceedings of the Second Conference on Machine Translation. 2017. aclanthology.org BT(Back Translation)과 BPE(Byte Pair Encoding)로 유명한 Rico Sennrich 교수팀이 WMT17에..
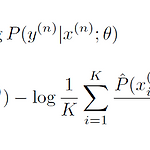
출처: https://dblp.org/rec/conf/aaai/WangXZBQLL18.html dblp: Dual Transfer Learning for Neural Machine Translation with Marginal Distribution Regularization. For web page which are no longer available, try to retrieve content from the of the Internet Archive (if available). load content from web.archive.org Privacy notice: By enabling the option above, your browser will contact the API of web.arch..
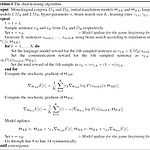
출처: https://arxiv.org/abs/1611.00179 Dual Learning for Machine Translation While neural machine translation (NMT) is making good progress in the past two years, tens of millions of bilingual sentence pairs are needed for its training. However, human labeling is very costly. To tackle this training data bottleneck, we develop a du arxiv.org 이번에 기계 번역기 구현 다 마치고, colab 환경에서 dual learning을 이용한 fine-..
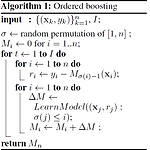
출처: https://arxiv.org/abs/1706.09516 CatBoost: unbiased boosting with categorical features This paper presents the key algorithmic techniques behind CatBoost, a new gradient boosting toolkit. Their combination leads to CatBoost outperforming other publicly available boosting implementations in terms of quality on a variety of datasets. Two criti arxiv.org 사실 이번에 개인적으로 진행하고 있는 대회에서 CatBoost를 상당히 ..
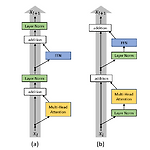
출처: https://arxiv.org/abs/2002.04745 On Layer Normalization in the Transformer Architecture The Transformer is widely used in natural language processing tasks. To train a Transformer however, one usually needs a carefully designed learning rate warm-up stage, which is shown to be crucial to the final performance but will slow down the optimizati arxiv.org 1. Introduction Transformer는 현재 자연어처리 외..
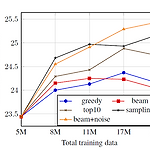
출처: https://arxiv.org/abs/1808.09381 Understanding Back-Translation at Scale An effective method to improve neural machine translation with monolingual data is to augment the parallel training corpus with back-translations of target language sentences. This work broadens the understanding of back-translation and investigates a numb arxiv.org 페이스북과 구글의 공동연구로 2018년 발표한 글입니다. 1. Introduction 기계번역기를..
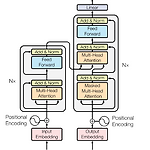
출처 : https://arxiv.org/abs/1706.03762 Attention Is All You Need The dominant sequence transduction models are based on complex recurrent or convolutional neural networks in an encoder-decoder configuration. The best performing models also connect the encoder and decoder through an attention mechanism. We propose a new arxiv.org 구글 연구팀에서 2017년 발표한 논문입니다. 구글의 기존 Seq2Seq 모델에 대응한 '페이스북의 ConvS2S'가 발표..
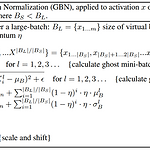
이스라엘 연구팀에서 2017년에 발표한 연구 논문입니다. Tabnet에서 사용된 Ghost BN에 대해서 찾아보다가 논문을 읽게 되어 리뷰해보려 합니다. Tabnet의 논문 리뷰는 이후에 진행하도록 하겠습니다. 1. Introduction 아직까지 인공지능 모델의 훈련에 있어 SGD는 중요한 역할을 하고 있습니다. 여담으로 Adam과 같이 Adaptive learning rate를 지원하는 방식은 훈련의 초기에 (특히 Transformer 아키텍처에서) 불안정한 양상을 보인더랬죠. 그럼으로 인해 SOTA급 성능을 뽑아내기 위해서 SGD 튜닝은 거의 불가피해 보입니다. 그러나 SGD의 특성상 국소 최적해에 빠지는 등 일반화의 문제에 대해서 많은 관심이 끌렸고, 그 중 하나가 배치 사이즈가 너무 크면 모델이..