목록논문 리뷰 (16)
while (1): study();
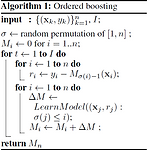
출처: https://arxiv.org/abs/1706.09516 CatBoost: unbiased boosting with categorical features This paper presents the key algorithmic techniques behind CatBoost, a new gradient boosting toolkit. Their combination leads to CatBoost outperforming other publicly available boosting implementations in terms of quality on a variety of datasets. Two criti arxiv.org 사실 이번에 개인적으로 진행하고 있는 대회에서 CatBoost를 상당히 ..
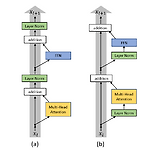
출처: https://arxiv.org/abs/2002.04745 On Layer Normalization in the Transformer Architecture The Transformer is widely used in natural language processing tasks. To train a Transformer however, one usually needs a carefully designed learning rate warm-up stage, which is shown to be crucial to the final performance but will slow down the optimizati arxiv.org 1. Introduction Transformer는 현재 자연어처리 외..
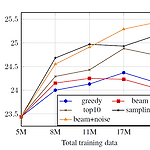
출처: https://arxiv.org/abs/1808.09381 Understanding Back-Translation at Scale An effective method to improve neural machine translation with monolingual data is to augment the parallel training corpus with back-translations of target language sentences. This work broadens the understanding of back-translation and investigates a numb arxiv.org 페이스북과 구글의 공동연구로 2018년 발표한 글입니다. 1. Introduction 기계번역기를..
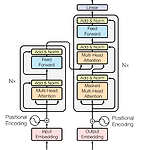
출처 : https://arxiv.org/abs/1706.03762 Attention Is All You Need The dominant sequence transduction models are based on complex recurrent or convolutional neural networks in an encoder-decoder configuration. The best performing models also connect the encoder and decoder through an attention mechanism. We propose a new arxiv.org 구글 연구팀에서 2017년 발표한 논문입니다. 구글의 기존 Seq2Seq 모델에 대응한 '페이스북의 ConvS2S'가 발표..
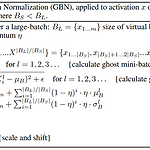
이스라엘 연구팀에서 2017년에 발표한 연구 논문입니다. Tabnet에서 사용된 Ghost BN에 대해서 찾아보다가 논문을 읽게 되어 리뷰해보려 합니다. Tabnet의 논문 리뷰는 이후에 진행하도록 하겠습니다. 1. Introduction 아직까지 인공지능 모델의 훈련에 있어 SGD는 중요한 역할을 하고 있습니다. 여담으로 Adam과 같이 Adaptive learning rate를 지원하는 방식은 훈련의 초기에 (특히 Transformer 아키텍처에서) 불안정한 양상을 보인더랬죠. 그럼으로 인해 SOTA급 성능을 뽑아내기 위해서 SGD 튜닝은 거의 불가피해 보입니다. 그러나 SGD의 특성상 국소 최적해에 빠지는 등 일반화의 문제에 대해서 많은 관심이 끌렸고, 그 중 하나가 배치 사이즈가 너무 크면 모델이..
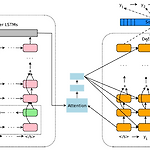
ArXiv 링크: https://arxiv.org/abs/1609.08144 Google's Neural Machine Translation System: Bridging the Gap between Human and Machine Translation Neural Machine Translation (NMT) is an end-to-end learning approach for automated translation, with the potential to overcome many of the weaknesses of conventional phrase-based translation systems. Unfortunately, NMT systems are known to be computationall..